Application
An image processing algorithm that differentiates between malignant and non-malignant cells in a hyperspectral image.
Key Benefits
- Able to detect head and neck cancer in hyperspectral images with higher than 95% accuracy and sensitivity.
- Allows for the identification of head and neck caner without an invasive procedure or expensive contrast agents.
Market Summary
The healthcare industry is undergoing a shift towards non-invasive diagnostics and therapeutic procedures. Technologies such as optical imaging have the potential to revolutionize clinical diagnostic safety. This technology uses a non-invasive procedure, without ionizing radiation or contrasting agents, which is less expensive than invasive alternatives. As such, optical imaging technologies are expected to continue growing as they increase their clinical acceptance. Hyperspectral imaging, originally developed for military and surveillance purposes, is now gaining traction as a new optical imaging technique for life science applications. This “full-spectrum” imaging technique has potential applications in stained slide microscopy, pathology identification, tumor margin detection for surgery, and non-invasive imaging disease diagnosis. Successful implementation of hyperspectral imaging technology will depend on accurate image processing algorithms that properly analyze the resulting complex data.
Technical Summary
Hyperspectral sensors collect information in a wide range of the electromagnetic spectrum. This range of electromagnetic information is then combined to form a three-dimensional data cube for processing and analysis. It has been reported that cancer cells and healthy cells have different electromagnetic signatures, which can be differentiated by hyperspectral imaging. However, classification of hyperspectral images is challenging due to the high spatial resolution, high dimensionality of spectral bands, and high redundancy caused by overlapping adjacent bands. In order to utilize both spectral and spatial information, Emory researchers have developed an algorithm, using a tensor-based modeling framework that is able to combine both the spectral and spatial data without having to alter the three-dimensional structure of the data.
Developmental Stage
This algorithm has been validated on hyperspectral images from four mice with head and neck tumors. It is able to detect tumors with higher than 95% accuracy and sensitivity.
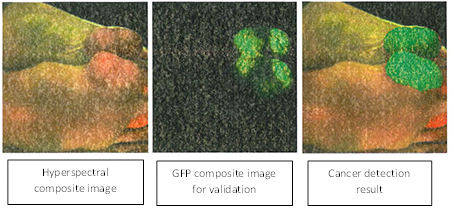
Read our featured innovation.